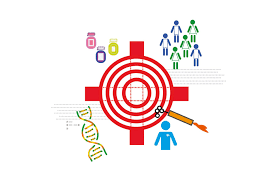
Application of AI in Precision Medicine
-Jeru M Manuel, Ph.D.
Precision medicine is a healthcare process that cumulatively applies multiple technologies to individually diagnose and treat patients based on their own biological make up [1]. This field is fast evolving, mainly due to the large-scale developments seen in ‘omics’ over the last decade. Owing to an improved understanding of the DNA with the completion of the human genome project at the dawn of this millennium, there has been a surge to explore its application in diverse fields. It is estimated that the field of health care would benefit the most, leading to an explosion in the development of genomics. Although precision medicine shows significant promise in improving population health, it is very much in infancy and there are its own challenges [2].
Over the last decade, the basis for personalizing health and lifestyle recommendations based on genomic information is one aspect of precision medicine which is gaining ground due to the off-shoot of many DTC companies. This preventative healthcare space encompassing the field of nutrigenomics is believed to offer risk traits based on single nucleotide polymorphisms (SNP’s-natural variations occurring in the genome), that with lifestyle modifications may have the potential to eventually reduce the burden of risk [3]. However due to the void in the number of studies, communicating the findings effectively using a single SNP approach is viewed with critical lenses. This is understandable, as biologically most functions occur with the interaction of multiple enzymes, which indicates the involvement of many genes. In addition to genetic factors, there is epigenetics and the microbiome which also contribute to final phenotype resulting in misinterpretation [4].
However, this is not of concern, it is very much similar to all other new concepts or innovations that have made their way. The hurdle in this process is mainly due to lack of efficient ways to translate the large research data into meaningful application for the population at large. Advances made in a similarly exciting field, Artificial intelligence (AI) offers promise [5]. AI works on the ability to train high performance computers with artificial learning algorithms to identify the variations in the multidimensional datasets that usually is impossible for a human to decipher. The core concept behind this is a set of computer algorithms that have the ability to identify patterns in large multidimensional datasets [6]. Applying learning strategies to predict the availability of similar patterns in other individuals, is put to use to predict and optimize the interpretation. Observations indicate that to identify polygenic risk scores, the promising approach for analysis of the results are by employing neural network driven machine learning algorithms in comparison to the older method of using polynomial algorithms [5-7].
DNA sequencing is the technology that has been at the forefront of genomics. The limitations are currently most of the genomics based technology only has the ability to short read genomic sequences []. Also there are no solutions to analyze the unknown risk contribution of repetitive regions and structural variations. Recently machine learning has been adopted to read long stretches of DNA fragments from digital electronic signalling data [5]. Long read technologies will be able to resolve the complexity of repetitive regions in the genome and detect complex structural variants. The nano-pore sequencing technology in particular has begun to use a neural network based deep learning method base calling the DNA sequence, this was seen to have and accuracy over 98% and can also produce mega base long DNA reads [8].
The other critical challenges associated with genomics are making functional sense out of the large data. This includes complexity in classifying mutations according to their clinical relevance due to the largely unknown penetrance of SNP’s [8,10]. Moreover low penetrance SNP’s are much more common than ones with higher penetrance. In addition most of these variants are non-coding in our genomes. Therefore, determining pathogenicity of rare or common non-coding variants still requires major advancement in genomics. Further-more many penetrant variants are also known to have more than one clinical manifestation, known as pleiotropy, and many diagnoses are characterized by variable presentation [11].
In line with all these requirements, attempts have been undertaken to use AI in the clinical classification of genomic variation. The ability of AI to analyze multidimensional biological data, and use of approaches will potentially help to decipher the pathogenicity. The basis for these learning algorithms include, analyzing the other contributory factors like characterization of non-coding variants, splicing code, DNA/RNA binding proteins and non coding RNA (ncRNA) using large scale molecular datasets [7,9-10,12]. It is therefore evident that application of AI in precision medicine holds significant promise for the future, and the field of preventative healthcare has the potential to revolutionize the perception of health.
- Sankar, P. L. & Parker, L. S. The precision medicine initiative’s all of us research program: an agenda for research on its ethical, legal, and social issues. Genet. Med. 19, 743–750 (2017).
- Athreya, A. P. et al. Pharmacogenomics-driven prediction of antidepressant treatment outcomes: a machine learning approach with multi-trial replication. Clin. Pharmacol. Ther.
Link (2019). - Vanessa Araujo Almeida et al. Comparison of Nutrigenomics Technology Interface Tools for Consumers and Health Professionals: A Sequential Explanatory Mixed Methods Investigation.J Med Internet Res, 21(6), 1-15 (2019).
- Consortium, E. P. An integrated encyclopedia of DNA elements in the human genome. Nature 489, 57–74 (2012).
- Padovani de Souza, K. et al. Machine learning meets genome assembly. Brief Bioinform. Link (2018).
- Kermany, D. S. et al. Identifying medical diagnoses and treatable diseases by image-based deep learning. Cell 172, 1122–1131 e1129.
- Alipanahi, B., Delong, A., Weirauch, M. T. & Frey, B. J. Predicting the sequence specificities of DNA- and RNA-binding proteins by deep learning. Nat. Biotechnol. 33, 831–838 (2015).
- Boza, V., Brejova, B. & Vinar, T. DeepNano: deep recurrent neural networks for base calling in MinION nanopore reads. PLoS ONE 12, e0178751 (2017).
- Wood, D. E. et al. A machine learning approach for somatic mutation discovery. Sci. Transl. Med. 10,
Link (2018). - Zhou, J. & Troyanskaya, O. G. Predicting effects of noncoding variants with deep learning-based sequence model. Nat. Methods 12, 931–934 (2015).
- Rizzi, R., Cairo, M., Makinen, V., Tomescu, A. I. & Valenzuela, D. Hardness of covering alignment: phase transition in post-sequence genomics. IEEE/ACM Trans. Comput. Biol. Bioinform. 16, 23–30 (2019).
- Xiong, H. Y. et al. RNA splicing. The human splicing code reveals new insights into the genetic determinants of disease. Science 347, 1254806 (2015).